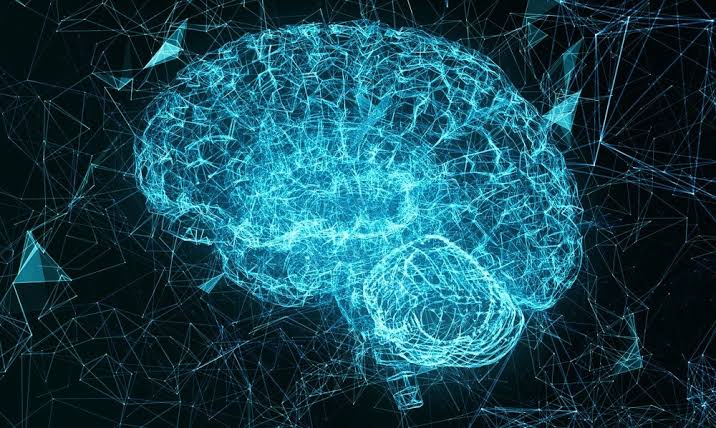
The field of AI and neuroscience have mutually benefited from each other’s principles. Today, researchers have validated AI models with the help of neuroscience. Similarly, deep neural network architectures have been developed with the assistance of biological neural networks. These architectures have numerous applications, such as speech recognition and text processing.
Neuroscientists have utilized AI-based systems to test their hypotheses and examine neuroimaging data, aiding in the early diagnosis and prediction of psychiatric disorders. Additionally, AI systems help establish a brain interface, send neurological signals, and develop commands for robotic arms.
AI’s ability to examine complex data and identify concealed patterns makes it an optimal choice for neuroscience data analysis.
The Design Of AI Systems Is Influenced By Neuroscience
Scientists designed recurrent neural networks (RNNs) inspired by the working memory function of the human brain. RNNs use past outputs as inputs to predict future outputs. One type of RNN, the long short-term memory (LSTM) network, can manage long-term dependencies, like those in text summaries.
Similarly, the architecture of the brain’s ventral visual stream inspired scientists to create convolutional neural networks (CNNs). In reinforcement learning (RL), intelligent computer agents comprehend the environment’s state and decide on actions. Moreover, computers learn to repeat specific tasks based on rewards and avoid others based on penalties.
Deep reinforcement learning (RL) uses neural networks to create a nonlinear mapping between the current environment state and all possible actions via gradient descent. Deep RL makes machine recognition of speech, text, and images possible. However, the technology requires significant computing resources.
In What Ways Is AI Applied In Neuroscience Research?
AI-assisted brain computer/machine interface (BCI) applications aid people with neuromuscular disorders like spinal cord injuries or cerebral palsy. Prosthetic control is also possible with AI, exemplified by BrainGate, an implant that enables users to control limb movements.
Also, neurological infections like meningitis are challenging to detect, given the diverse symptoms. Nevertheless, AI-based approaches could accurately forecast meningitis type using predictor variables like lymphocytes, cerebrospinal fluid (CSF) neutrophils, and neutrophil-to-lymphocyte ratio (NLR). AI has several benefits when applied to neuro-oncology, providing precise initial diagnoses and therapeutic options.
Featured image from towardsai.net