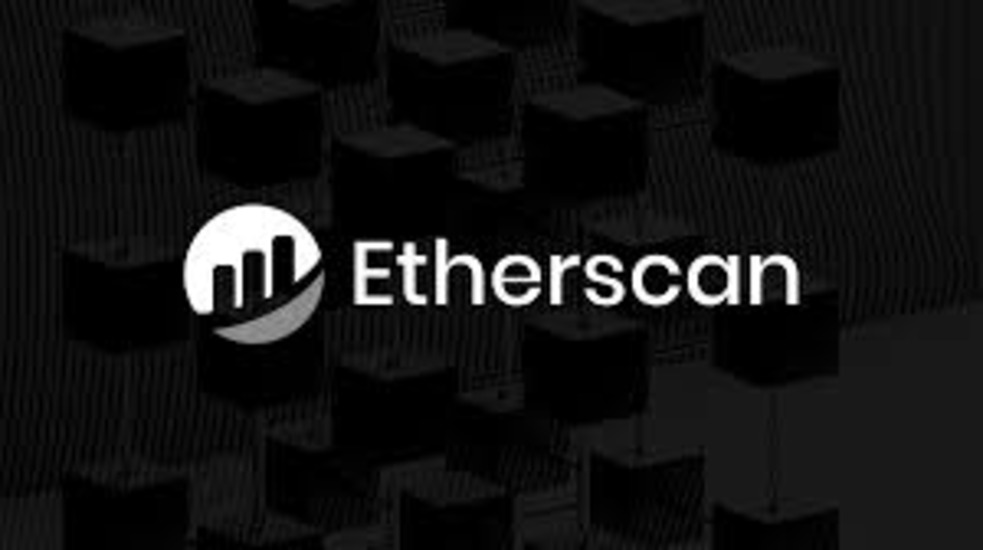
Etherscan recently introduced a tool called “Code Reader.” The tool leverages AI to fetch and interpret a contact address source code. Users can generate responses using OpenAI’s language model by entering a prompt. It provides users with useful information about the contract’s relevant source code.
Code Reader Usages
The capabilities of Code Reader are diverse and innovative. It empowers users to access extensive lists of Ethereum data-related smart contract-related functions, expanding their understanding of the contract’s capabilities. The tool can decipher the intricate relationship between the contract and decentralized applications.
Upon retrieving the files, Code Reader allows users to select and explore specific files of the relevant source code. The tool’s user-friendly interface facilitates direct modifications to the source code. This unique functionality empowers users to tailor the code to their needs before sending it to AI.
Following the unveiling of a filter tool on 2nd June 2023, Etherscan introduced an AI-powered tool that complements its functionality. These tools enhance data analysis for researchers, on-chain detectives, and investigators. This is possible with AI capabilities and a diverse array of new search functions. The year 2023 has witnessed the emergence of AI concepts within the blockchain and cryptocurrency world.
Experts Concerns
However, with the ongoing AI boom, experts have raised valid concerns regarding how feasible existing AI models are. A recent Foresight Ventures report emphasizes that computing power resources will become the next central battleground. Big AI model training places a greater burden on distributed computer networks. Yet, researchers recognize that current prototypes have serious shortcomings. These limitations encompass challenges related to network optimization and concerns surrounding security and privacy.
Foresight researchers illustrated that the training of a massive model containing 175 billion variables requires approximately 700 gigabytes of storage. However, these parameters must get updated regularly between multiple computing nodes when employing distributed training.
The featured image is from coinmarketcap.com